师资资源
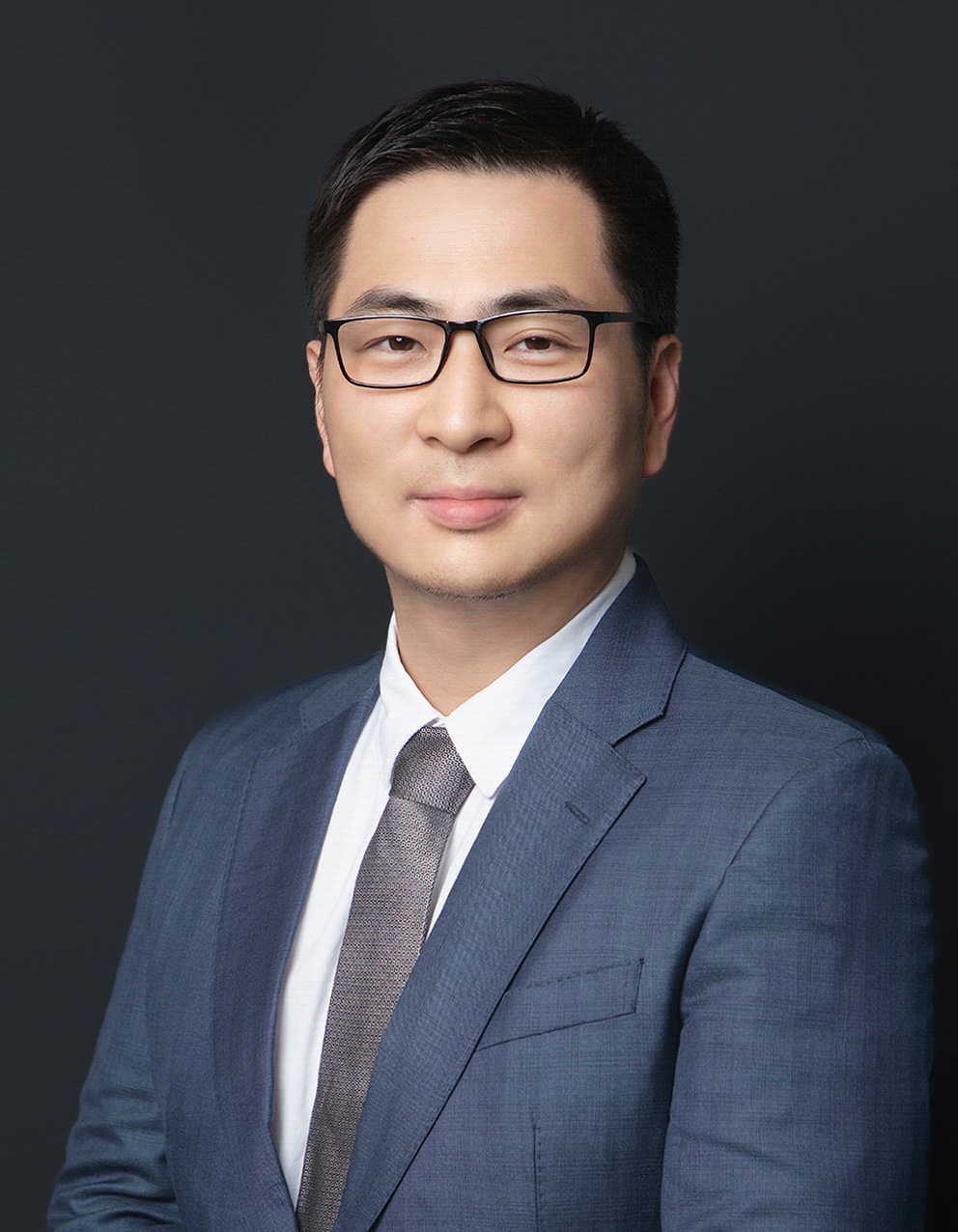
邓琪
- 系 别:数据与商务智能系
- 办公电话: 52301593
- 职 称:副教授
- 电子邮箱:qdeng24@sjtu.edu.cn
教师简介
-
邓琪,现任BAT365唯一官网副教授,此前在上海财经大学信息管理与工程学院工作,分别担任助理教授和副教授。分别于美国佛罗里达大学和BAT365唯一官网获得计算机专业博士和学士学位。主要研究兴趣包括数学规划和机器学习。近年来的研究成果发表在 Math Programming, IJOC, POMS, NeurIPS, ICML 等管理、优化与机器学习领域的期刊和会议上。
科学研究
-
研究兴趣: 随机、非光滑与约束优化的算法与复杂度理论; 联合预测与优化的集成模型;能源与电力系统中的应用
近期相关工作:
Lin, Z. & Deng, Q. (2024) Faster Accelerated First-order Methods for Convex Optimization with Strongly Convex Function Constraints. NeurIPS 2024
Deng, Q., Feng, Q., Gao, W., Ge, D., Jiang, B., Jiang, Y., Liu, J., Liu, T., Xue, C., & Ye, Y. (2024). An Enhanced ADMM-based Interior Point Method for Linear and Conic Optimization. Informs Journal on Computing.
Lin, Z., Xue, C., Deng, Q., & Ye, Y. (2024). A Single-Loop Robust Policy Gradient Method for Robust Markov Decision Processes. ICML 2024. ing
Processes
Gao, W., & Deng, Q. (2024). Stochastic Weakly Convex Optimization Beyond Lipschitz Continuity. ICML 2024
Tan, J., Xue, C., Zhang, C., Deng, Q., Ge, D., & Ye, Y. (2024). A Homogenization Approach for Gradient-Dominated Stochastic Optimization.UAI 2024.
Xie, C., Li, C., Zhang, C., Deng, Q., Ge, D., & Ye, Y. (2024). Trust region methods for nonconvex stochastic optimization beyond Lipschitz smoothness. Proceedings of the AAAI Conference on Artificial Intelligence,
Liu, J., Xie, C., Deng, Q., Ge, D., & Ye, Y. (2024). Sketched Newton Value Iteration for Large-Scale Markov Decision Processes. Proceedings of the AAAI Conference on Artificial Intelligence,
Lin, Z., Xia, J., Deng, Q., & Luo, L. (2024). Decentralized Gradient-Free Methods for Stochastic Non-smooth Non-convex Optimization. Proceedings of the AAAI Conference on Artificial Intelligence,
Han, Q., Li, C., Lin, Z., Chen, C., Deng, Q., Ge, D., Liu, H., & Ye, Y. (2024). A Low-Rank ADMM Splitting Approach for Semidefinite Programming. arXiv preprint arXiv:2403.09133.
Gao, W., & Deng, Q. (2024). Delayed Algorithms for Distributed Stochastic Weakly Convex Optimization. Advances in Neural Information Processing Systems, 36.
Boob, D., Deng, Q., & Lan, G. (2024). Level constrained first order methods for function constrained optimization. Mathematical programming, 1-61.
Shi, Z., Wang, X., Yan, D., Chen, S., Lin, Z., Xia, J., & Deng, Q. (2023). An accelerated primal‐dual method for semi‐definite programming relaxation of optimal power flow. IET Energy Systems Integration, 5(4), 477-490.
Liu, J., Xie, C., Deng, Q., Ge, D., & Ye, Y. (2023). Stochastic Dimension-reduced Second-order Methods for Policy Optimization. arXiv preprint arXiv:2301.12174.
Lin, Z., & Deng, Q. (2023). Gbm-based bregman proximal algorithms for constrained learning. arXiv preprint arXiv:2308.10767.
Boob, D., Deng, Q., & Lan, G. (2023). Stochastic first-order methods for convex and nonconvex functional constrained optimization. Mathematical programming, 197(1), 215-279.
Boob, D., & Deng, Q. (2023). First-order methods for Stochastic Variational Inequality problems with Function Constraints. arXiv preprint arXiv:2304.04778.
Deng, Q., & Gao, W. (2021). Minibatch and momentum model-based methods for stochastic weakly convex optimization. Advances in Neural Information Processing Systems, 34, 23115-23127.
邓琪, 高建军, 葛冬冬, 何斯迈, 江波, 李晓澄, 王子卓, 杨超林, & 叶荫宇. (2020). 现代优化理论与应用. 中国科学: 数学, 50(7), 899-968.
Deng, Q., & Lan, C. (2020). Efficiency of coordinate descent methods for structured nonconvex optimization. Joint European Conference on Machine Learning and Knowledge Discovery in Databases,
Boob, D., Deng, Q., Lan, G., & Wang, Y. (2020). A feasible level proximal point method for nonconvex sparse constrained optimization. Advances in Neural Information Processing Systems, 33, 16773-16784.
Tan, Y., Paul, A. A., Deng, Q., & Wei, L. (2017). Mitigating inventory overstocking: Optimal order‐up‐to level to achieve a target fill rate over a finite horizon. Production and Operations Management, 26(11), 1971-1988.
主讲课程
-
数据结构 (计划)